by Morten Jerven Associate Professor, Simon Fraser University, Canada
On 5 November 2010, Ghana Statistical Services announced that it was revising national GDP estimates upwards by over 60 percent. After the revision a range of new activities, worth about US$13 billion, were accounted for, and Ghana was thus upgraded from a low-income country to a lower-middle-income country. In the fall of 2011 its near-neighbor Nigeria also announced a forthcoming revision of its GDP. This revision is not yet complete, but it has been suggested that the GDP revision in Nigeria will cause a similarly large jump in GDP. If GDP doubles in Nigeria following the revision it will mean that the GDP for the whole region increases by more than 15 percent. The value of the increase amounts to as much as 40 economies roughly the size of Malawi’s.
The revisions have caused confusion and disbelief in the development community. In response to a paper I presented in South Africa in 2011, laying out the size and extent of the revision, Shanta Devarajan declared it: Africa’s Statistical Tragedy (1, 2).
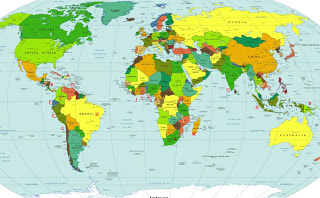
The cause for these large upward revisions is that the base year, the foundation of the system that generates GDP numbers had not been updated in Nigeria and Ghana for over two decades. As I report in my book (Poor Numbers: How We Are Misled by African Development Statistics and What to Do about It), there are many countries that are in a similar position (3). Huge discrepancies and alarming gaps in statistical knowledge exist about productivity, income distribution, and population and any ranking of African economies according to GDP is misleading.
A short history of measuring progress in Africa
In Africa, as elsewhere, income statistics need to be fully historicized and contextualized to be understood. Though these data are often presented as facts, they are better considered as products, whose production is subject to substantial economic and political constraints. The statistical capacity of African states was greatly expanded in the late colonial and early postcolonial period, but it was greatly impaired during the economic crisis of the 1970s. In the decades of policy reform that followed—the period of “structural adjustment” in the 1980s and 1990s the importance of statistical offices was neglected. For statistical offices, structural adjustment meant having to account for more with less at a time when informal and unrecorded markets were growing and public spending was curtailed. In retrospect it is puzzling that the International Monetary Fund (IMF) and the World Bank embarked on growth oriented reforms without ensuring that there were reasonable baseline estimates that could plausibly establish whether the economies were growing or stagnating. Regardless, the consequence is that our knowledge about the economic effects of structural adjustment is limited. More generally, the economic growth time series, or the cumulative record of annual growth between 1960 and today does not appropriately capture changes in African economic development.
Controversy: lessons from the datasets
Economists commonly ask: “Yes, we know that there are measurement problems. But does it really matter?” The short answer is yes: policymakers, nongovernmental organizations, and scholars draw different conclusions based on the different datasets they use in their analysis. Numbers currently used to allocate scarce resources are not good enough for these purposes; equally the econometric models we currently use to explain differences in development performance are far more impressive than the numbers they set out to explain. In Poor Numbers, I present some numerical examples, like the case of Malawi discussed below, that underscore the dangers of ignoring data quality.
For example, many African nations struggle with the problems of population censuses, with how to capture the informal sector, and with agricultural statistics. Because these data are assembled using competing methods and assumptions, the final data series are subject to political pressure, particularly when the government is subsidizing agricultural inputs. One example is Malawi. The most recent agricultural census (2006/2007) indicated a maize output of 2.1 million tonnes, compared to the previously widely circulated figures of 3.4 million tonnes from the Ministry of Agriculture. Specifically, the re-introduction of Malawian agricultural subsidies from 2005 onwards created a persistent political demand for high growth rates. What seems to have happened is that the demands for high growth rates were spontaneously met in collusion by farmers and agricultural officers who oversubscribed to fertilizer vouchers, thus creating an illusion of growth in the Ministry of Agriculture annual data. This ‘Malawi Miracle’ has been widely touted by leaders of the UN’s Millennium Project and Jeffrey Sachs wrote in the New York Times: “African leaders, such as President Bingu wa Mutharika of Malawi, also broke old donor-led shibboleths by establishing new government programs to get fertilizer and high-yield seeds to impoverished peasant farmers who could not afford these inputs. Farm yields soared once nitrogen got back into the depleted soils.” (4). Undoubtedly, there have been improvements in production in Malawi, but the success is much overstated in the official statistics. In 2007, if the official statistics had been correct, food prices should have been dropping, or Malawians should be putting on weight or Malawi should have exported a lot of maize – yet none of these things occurred. The case shows how weak statistical systems may respond when there are clear political and financial rewards for adjusting the numbers.
When designing systems for providing agricultural input subsidies this effect on the statistical system must be closely watched, otherwise the reality and the numerals can quickly depart. Discrepancies such as those found here, in the order of plus/minus 30 percent can be vital differences in countries where a large part of the population is living in, or close to, absolute poverty.
What to do about it?
Using survey information and interviews conducted in field research, it is possible to build a picture of the current situation at statistical offices in sub-Saharan Africa.The current development agenda is set by the Millennium Development Goals of the United Nations. This has led to some statistical capacity building in a number of countries, while in others there have been perverse effects when statistical capacity is diverted to data collection in order to monitor particular donor targets. At present there is no coherent global strategy for improving the provision of data for development and I argue that putting a coherent global strategy for statistical capacity building in place is important. There is currently a big demand for data, but so far it has not been well thought through how these data are to be supplied. Any development agenda must to be geared toward solving local problems, and this needs to include statistical capacity. The book advances several useful and practical steps toward such an agenda.
These issues are related to the general problem of accountability in development. Sometimes ignorance is bliss for both the donor community and local political leaders. Poor numbers give room to both politicians and donors that want to negotiate numbers upwards or downwards. It is not always that better data is ‘better data’. As I document in the book, trends in poverty and agricultural production can appear much better in the administrative data (those collected by the government) than those collected in surveys. Consequently, some of the current growth data paints a too optimistic picture of what is happening to Africa. In particular, there are serious gaps in the data that should make us far more cautious in asserting that the current episode of growth is ‘inclusive’. As indicated, some of this very high growth is driven by upward revisions, at the same time, a lack of data on poverty in many of the big countries (Nigeria, Sudan, Angola, DRC), as well as missing data from the poorer countries on the continent means that better data would probably paint a more sobering picture than the current very optimistic narratives. For example, Sala-i-Martin and Pinkovskyi claim that poverty in Africa is falling very quickly. Their findings are based, however, on an investigation that extrapolates poverty data from small rich countries in Africa to poor big poor countries; and since there are very few observations since 2002 in their sample, poverty reduction is assumed to grow in line with GDP – which we know is a) overstated in recent years and b) not the same as a reduction in poverty. Many other current accounts are dealing with the data availability and data quality problem in a deeply unsatisfactory way. We need to acknowledge that there is a knowledge problem, and that our knowledge is doubly biased. We know less about poor countries, and we know less about the poor people in those poor countries.
Conclusion
The state of the scholarly literature today, particularly in African studies and International development, and more generally in the social sciences, is deeply unsatisfactory. The literature has either neglected the issue of data quality and therefore accepted the data at face value or dismissed the data as unreliable and therefore irrelevant. The truth is more complex: that current estimates are doubly biased in that we know less about those economies that are poorer, and less about the populations that are poor in those poor economies.
This is an encouragement to further scholarship. Numbers should and will continue to be important for the study and practice of development. Decisions are made because of these numbers; histories of development are written with these numbers. Scholars who already are critical of how numbers are used in both scholarship and policy should use their critical skills to further engage with the numbers. Decisions about what to measure, who to count, and by whose authority the final number is selected do matter. I hope this book leaves us better equipped to take on the task of taking part in these decisions, because poor numbers are too important to be dismissed as just that.
Footnotes
1. African Development Bank, Statistics Department. (2013). ‘Situational Analysis of Economic Statistics in Africa: Special Focus on GDP Measurement’.
Devarajan, S. (2013). ‘Africa’s Statistical Tragedy’. Review of Income and Wealth, DOI 10.1111/roiw.12013.
2. International Monetary Fund. (2013). ‘Sub-Saharan Africa: Building Momentum in a Multi-Speed World’.
3. Jerven, M. (2013a). Poor Numbers: How We Are Misled by African Development Statistics and What to Do about It. Ithaca, NY: Cornell University Press.
4. —— (2012a). The Political Economy of Agricultural Statistics and Input Subsidies: Evidence from India, Nigeria and Malawi, Journal of Agrarian Change, DOI: 10.1111/joac.12025
References
—— (2013b). ‘For Richer, for Poorer: GDP Revisions and Africa’s Statistical Tragedy’. African Affairs, 112(446): 138–147.
—— (2012). ‘Comparability of GDP Estimates in Sub-Saharan Africa: The Effect of Revisions in Sources and Methods since Structural Adjustment’. Review of Income and Wealth, DOI: 10.1111/roiw.12006.
—— (2010a). ‘The Relativity of Poverty and Income: How Reliable Are African Economic Statistics?’. African Affairs, 109(434): 77-96.
—— (2010b). ‘Random Growth in Africa? Lessons from an Evaluation of the Growth Evidence on Botswana, Kenya, Tanzania and Zambia, 1965–1995’. Journal of Development Studies, 46(2): 274-94.
Sala-i-Martin, X., and M. Pinkovskiy. (2010). ‘African Poverty is Falling…Much Faster than You Think’. NBER Working Paper 15775. Cambridge, MA: National Bureau of Economic Research.
Sandefur J. and A.Glassman: The political economy of bad data: Evidence from African survey & administrative statistics, Paper presented at UNU-WIDER Development Conference: Inclusive Growth in Africa Measurement, Causes, and Consequences, 20-21 September 2013
If this article was useful to you please consider sharing it with your networks.
Further reading:
The Not-So-Great Professor: Jeffrey Sachs’ Incredible Failure to Eradicate Poverty in Africa is reviewed here
http://www.psmag.com/culture/smart-guy-jeffrey-sachs-nina-munk-idealist-poverty-failure-africa-65348/